Graduate Research Symposium 2023
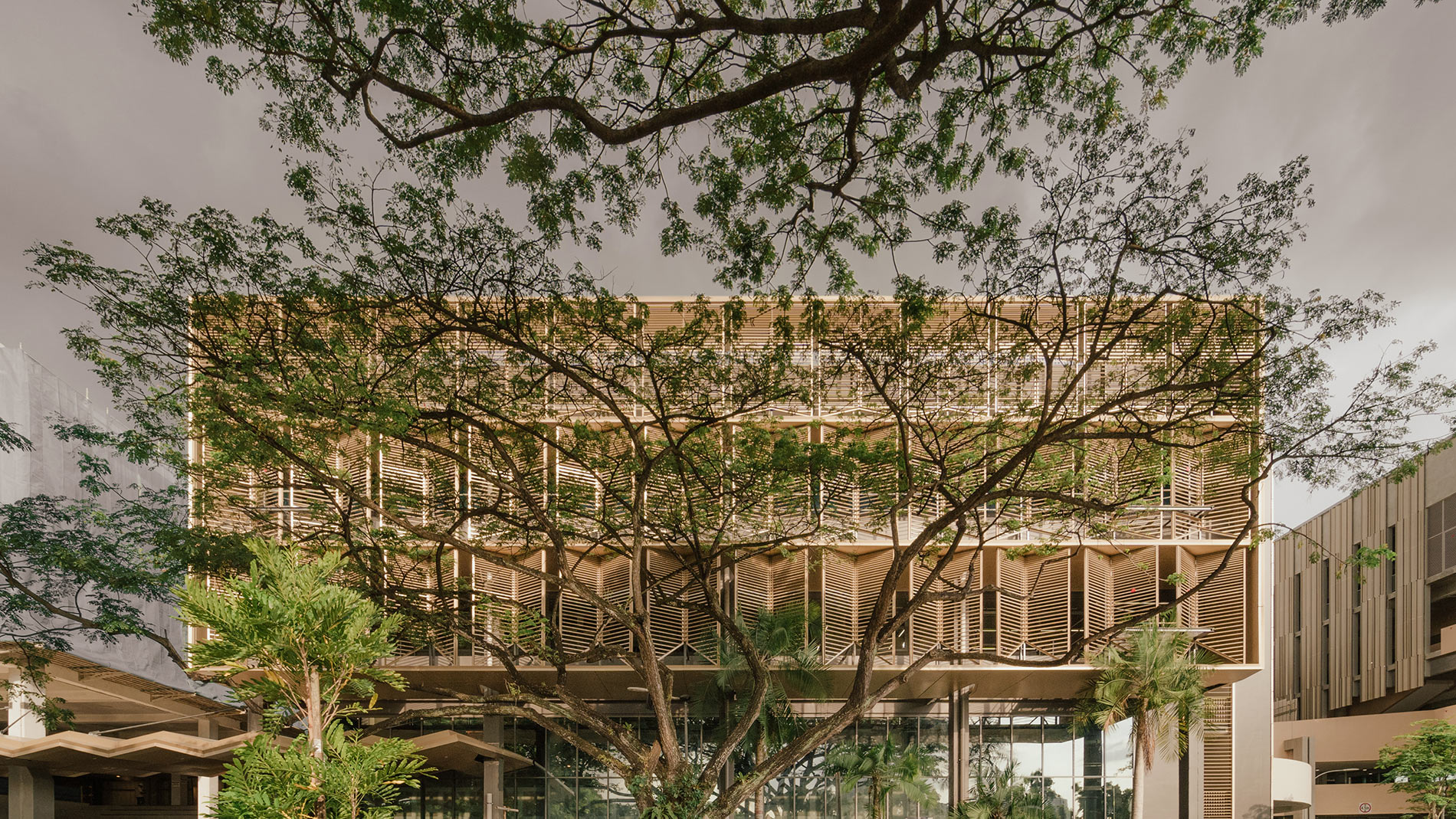
Built Environment Modeling 1
Time: 12:00PM – 13:40 PM
Venue: Seminar Room 08, SDE3 Floor4
Reviewers: Yuan Chao and Florian Heinzelmann
Speakers:
ZICHENG FAN
LEARNING URBAN NIGHT-TIME LIGHTING LANDSCAPE WITH STREET VIEW IMAGES
Abstract: Urban lighting plays a crucial role in facilitating night-time activities and serves as a lens to study human behaviour. Traditional studies often use satellite-captured night-time lights images (NTL) as proxies for human activity patterns like urban economic vitality and energy consumption. However, these approaches lack fine-grained detail and are dependent on satellite image availability. There is currently an absence of studies to directly measure the night-time lighting landscape from a human perspective, and to investigate how the lighting landscapes and the human activities are interacted with each other.
This study fills the gap by leveraging street view images (SVI), which are increasingly used for understanding urban environments due to their low data cost and rich content. The project explores the multitemporal potential of SVI as day-night paired sensors, and hope to investigate to what extent we can predict the luminosity and lighting landscape of a night-time urban scenario based on its corresponding day-time SVI.
Utilizing 1863 day-night SVI pairs from Singapore, we initially identify the 3D distribution and luminosity intensity of lighted areas as the lighting landscape, and apply k-means clustering method to distinguish different night-time urban patterns. On this basis, deep learning models, built on the EfficientNet architecture are trained to predict night-time luminosity and lighting patterns based on corresponding day-time SVI.
According to our exploration, there are 5 distinct lighting patterns revealed in the night-time SVI, which correspond to different urban function scenarios and road levels. And the luminosity prediction model also shows satisfying performance. The study confirms the reliability of using day-time SVI for night-time lighting analysis, offering a significant contribution to cross-modal information mining in urban studies.
KOICHI ITO
EXAMINING THE CAUSAL IMPACTS OF THE BUILT ENVIRONMENT ON ACTIVE TRANSPORTATION USING TIMESERIES STREET VIEW IMAGERY
Abstract: The development of computer vision techniques and the expansion of street view imagery (SVI) coverage has enabled researchers to examine the associations of street features with active transport patterns at a spatially fine and large scale. However, no study has taken advantage of spatiotemporal distributions of street features extracted from SVI to investigate the causal impacts of street features on active transport; thus, it has remained unclear whether certain street features actually affect cyclists and pedestrians.
To fill this research gap and gain more policy-relevant insights, this study utilized the historical SVI
data and cyclist count data in London and pedestrian count data in Montreal to infer causal impacts of vegetation, slope, and other street infrastructures on the number of cyclists and pedestrians with propensity score matching models and causal forest models.
Through analysis, we identified the following insights: 1. vegetation has significantly positive effects on cycling while it does not have significant effects on walking, 2. slope has significantly negative effects on cycling and significantly positive effects on walking activities, and 3. bike lane and sidewalk have significantly positive effects for cycling and walking, respectively. Moreover, the importance of causal inference methods and heterogeneous treatment effects were also identified.
This study went beyond simple correlations and inferred causal impacts of urban design on active transport activities, which is essential in understanding the actual effects of policy interventions. Therefore, this study’s findings are valuable for urban planners and designers to make cities more bikeable, walkable, and sustainable. Future studies can extend this study by implementing more experimental research, investigating more visual features, and adding more diverse study sites.
FRANCESCO TIZZANI
CIRCULAR CONSTRUCTION
Abstract: Construction is often associated with the sole Permanent Works (PW) that need to be constructed, leaving behind all those activities that are necessary to support the construction of PW. These activities fall into the definition of Temporary Works (TW), representing a significant part of any construction project, sometimes 50\% or more of the construction’s cost.
Because of its significant impact on the construction process, we think that TW could be a particularly valuable use case to study the implementation of Circular Construction (CC) approaches related to designing and reusing materials and components in the TW process. Reuse practices may already take place on many construction sites, but currently, there are no standard or CE-centric approaches. This paper introduces a high-level framework to define the system of three core tasks and technologies to enable Temporary Works’ Circularity: data collection standards, data capturing methods, and a digital TW trading platform.
Firstly, we evaluate what data needs to be captured for material assets, and the potential applicability of Materials Passports. Secondly, we discuss how this data can be collected through current TW’s procedures, based on examples from the industry. Thirdly, we map out the requirements for a digital environment that allows for contract-based trading of TW materials linked to project timelines to inform TW designers on what is available, when and where, and influence their design based on resource availability.
Due to the nature of the TW and the relatively short life span of these works, ranging between a few weeks to a few years, we propose that a system following our framework architecture would allow for verification and testing of CC approaches on TW’s miniature Life Cycle, and can help define possible leverage points and set the direction for a wider implementation of CC throughout the life cycle of PW.
ABRAHAM NOAH WU
A WORLD IMAGINED BY AI – GENERATING SPATIAL DATA USING GENERATIVE ALGORITHMS
Abstract: High quality of geospatial data, such as highly accurate terrain models, up-to-date location signals, or high resolution aerial images, are the bread and butter of data driven urban research and GIS. However, the access to high quality geospatial data is not shared equally. Regions with high quality GIS data in one domain may be entirely lacking in data for another application. Or in some cases, a region might still be lacking in any kind of high quality GIS data, and still relies on coarse, incomplete and mostly out-dated data. We define this phenomenon of uneven distribution and access of high quality geospatial data as Geospatial Data Asymmetry (GDA), and have found that existing GIS data collection and processing methods perpetuate this asymmetry.
In this thesis, we propose a new methodology to balance the data asymmetry using generative algorithms. This method neither relies on sensors nor on the expert knowledge of GIS professionals which were the cause of the GDA in the first place. Instead, it is able to transform lower quality data of a location into high quality data as long as there are already examples of high quality data collected in other locations.