Graduate Research Symposium 2023
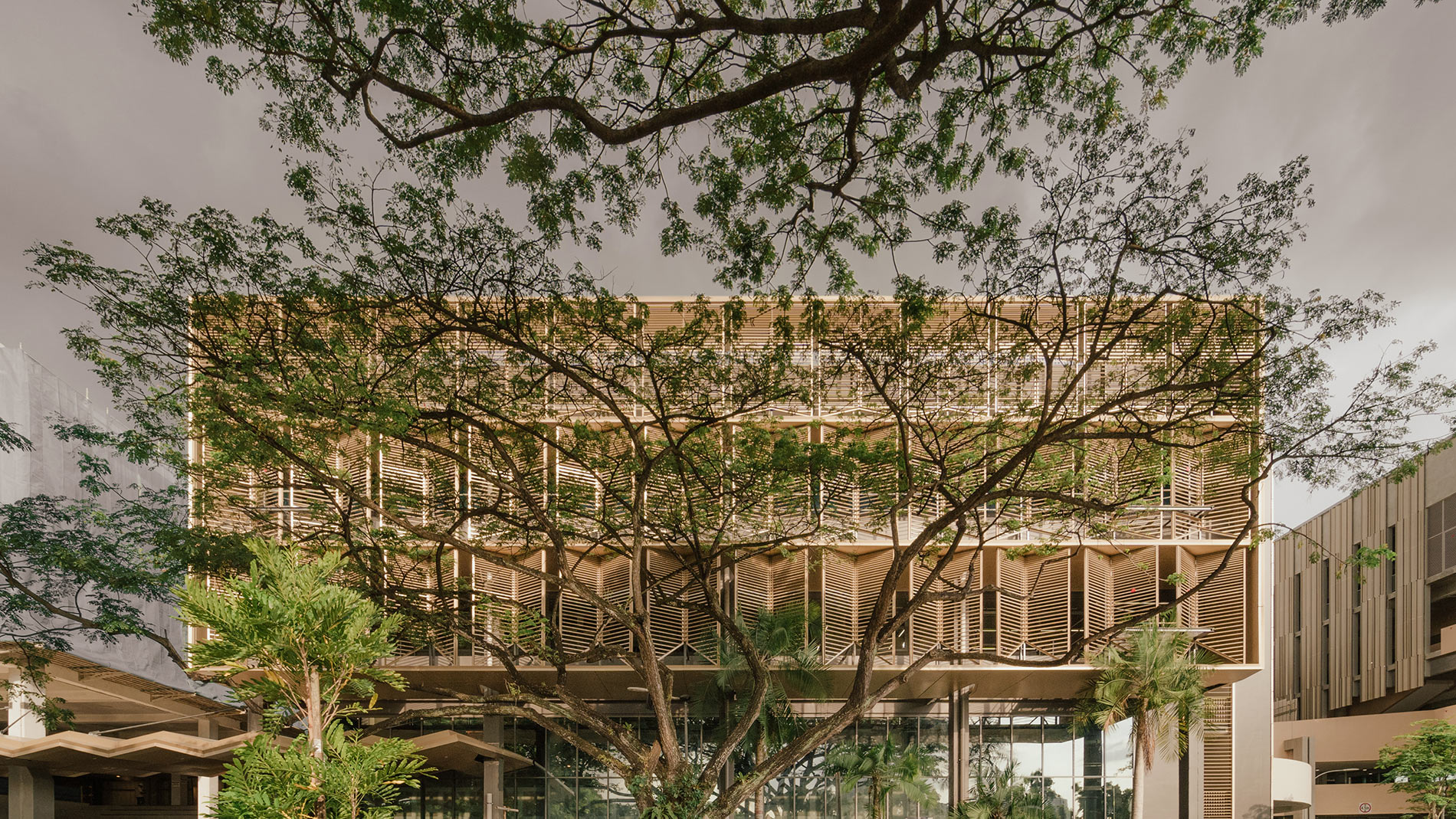
Urban Design and Human Perspective
Time: 15:00PM – 16:40 PM
Venue: Seminar Room 07, SDE3 Floor4
Reviewers: Ho Puay Peng
Speakers:
CAO QIAN
EVALUATING AI-GENERATED DESIGN SCHEMES: UNCOVERING INDICATORS FOR ENHANCED QUALITY
Abstract: AI technology has ignited a revolution across various industries, particularly in the building and construction sector, owing to its inherent “creativity.” Research groups have introduced AI applications like Delve, Giraffe, ARCHITECTURES, HyperAI, PlanFinder, XKool, Noah, and EvoMass, which automatically generate design schemes to attain multi-objective optimization based on given targets. These AI applications heavily rely on training databases, akin to the knowledge and experiences of senior designers.
However, many designers remain sceptical of design schemes produced by AI, contending that this approach is still in its nascent and unsatisfactory stages. Urban and architectural design entails intricate problem-solving processes, encompassing quantifiable indicators that can serve as constraints for AI-generated output. Nevertheless, certain indicators defy quantification and definition. While AI technology holds immense promise, our focus should concentrate on refining it to support designers, rather than resisting its implementation.
This study critically evaluates AI-generated design schemes from the perspectives of users and designers, identifying indicators overlooked in AI generation but significantly affecting the design quality to enhance AI performance. To achieve the research objective, this study employs a hospital design project as a case study. Such a project requires comprehensive and meticulous consideration, as it must fulfil multiple objectives, including sunlight exposure, natural ventilation, and emergency evacuation. Two design schemes generated by EvoMass are compared with a design scheme created by experienced designers. This comparison is conducted through a VR experiment and an eye-tracking experiment, in order to record and analyze the subjective and objective reactions of both users and designers.
In the VR experiment, participants are tasked with two activities, yielding quantitative assessments based on their performance. The first task involves identifying the hospital while navigating the surroundings and locating the main entrance. The second task entails locating the entrance of the inpatient building after they enter the hospital. Participants, categorized as professionals with a background in architecture and urban design or non-professionals without relevant discipline experience, will be assessed based on task completion status, time taken, and distance covered. Post-experiment interviews will also be conducted.
The eye-tracking experiment requires participants to assess and determine which design scheme closely resembles the work of senior designers from a set of images of three design schemes. Participants are divided into professional and non-professional groups according to their backgrounds. The experiment presents the general plan, aerial view, and human-eye view perspective of the three design schemes on a screen. Both quantitative eye-tracking data and qualitative verbal feedback will be collected for subsequent analysis.
During the data analysis phase, attention is directed toward disparities between professional and non-professional evaluations of the design schemes. Professionals are more concerned about the relationship between the building and the site than non-professionals. Although presently underemphasized, this topological relationship of outdoor spaces holds significance, particularly for large public buildings. This study introduces a new perspective to the exploration of AI-generated design and its results offer valuable insights for enhancing AI-generated outputs.
LI WENPEI
DATA-DRIVEN STUDY ON URBAN GREENSPACES AND PHYSICAL ACTIVITY IN TROPICAL HIGH-DENSITY CITY
Abstract: In response to the global issue of physical inactivity and associated chronic diseases, the World Health Organization has proposed optimizing the environment to improve public health. Urban greenspaces (UGS) constitute crucial components of urban environments with the capacity to facilitate physical activity (PA), yielding manifold benefits. Extensive studies have investigated the determinants of PA, including the micro UGS features, given the challenges associated with augmenting UGS in compact cities. Nevertheless, traditional approaches measuring UGS features and PA like onsite observations and surveys, offer insights but suffer from high expenses, subjectivity, and spatiotemporal biases. Big data is a rich source of spatiotemporal data, capable of yielding extensive insights into UGS features and PA on a broader scale at the population level. However, scant research delves into UGS features and PA by using big data, particularly textual data, which offer richer user experiences than images. Moreover, current UGS research favors unsupervised approaches for processing texts, despite supervised approaches often outperforming in most contexts. Addressing these gaps, this study aims to develop an unsupervised Natural Language Processing (NLP) framework and a supervised NLP framework. In the context of a densely populated tropical city characterized by significant physical inactivity and abundant UGS, namely Singapore, this study utilizes two NLP frameworks to explore the connections between UGS features and PA through online reviews. Mobility data and survey responses are concurrently utilized as measurements for PA.
Simultaneously, the study sheds light on the advantages and challenges inherent in each framework by comparing results from two frameworks. The two NLP frameworks, alongside the outcomes of the comparative analysis, offer valuable benchmarks for researchers. Additionally, the results obtained from the unsupervised NLP framework will complement and enhance the supervised NLP framework. Ultimately, the insights garnered regarding the relationships between UGS and PA will furnish UGS planners with instructive guidelines to enhance strategies aimed at promoting UGS-based PA.
LI SIYU
HEATWAVES AND HUMAN SENTIMENT: A GEO-TEMPORAL ANALYSIS USING SOCIAL MEDIA DATA
Abstract: More and more extreme heatwave events occur with global warming, which contributes to an intensified impact on various aspects of human life. Geo-tagged social media data can be collected from social media platforms such as Twitter and Instagram in terms of geographic spread (location) and temporal dynamics (time). They can present an amount of valuable information for understanding public sentiment and response to these events.
While previous studies have mined social media for insights into public sentiment for case studies, there is a gap in the comprehensive analysis that considers both spatial and temporal sentiment interactions of heatwave events. This research bridges this gap by examining geo-tagged social media posts in relation to extreme heatwave occurrences. The study revolves around three primary objectives. First, investigate the spatial patterns of social media posts linked to heatwave and extreme heatwave events. Second, explore trends in social media activity before, during, and after the extreme heatwave events. Third, compare social media data with the extreme heatwave events occurrence to understand variations in public response and sentiment across different regions.
In conclusion, this study lays the groundwork for future interdisciplinary research.
TANYA TALWAR
FRAMEWORK FOR IDENTIFYING HEATWAVES AND ASSESSING THEIR IMPACT IN A TROPICAL HIGH DENSITY CITY
Abstract: Climate extremes are of substantial significance to resilience studies because of their high short-term and long-term impact on economic, human, ecological, and urban-physical systems. Weather anomalies lead to high temperatures, fires, droughts, flash floods and their compound events. Most recently, in April 2023, several heat waves began across parts of the northern hemisphere causing severe damage. Singapore recorded the highest daily maximum temperature of 37 deg C in May 2023 in last 40 years and temperature breached 36 deg C across several parts of the island that month. According to studies on climate analogues, cities in tropical regions will face unprecedented climate extremes, prompting a need for preparedness for heat scenarios that have not yet been experienced elsewhere in the world.
Additionally, the urban population is at a greater risk of high temperatures due to the urban heat island effect. Studies conducted in multiple states of US show synergies between UHI and heatwaves and that the former amplifies the risk of latter. In this study, we use Singapore as a case to examine a suitable framework for identifying heatwaves in a tropical city and assessing their impact on the urban microclimate. We review the existing methodologies in place for defining and measuring heatwaves and investigate their suitability for a hot humid context. Further, we inquire into the mitigation and adaptation measures currently in place for the vulnerable population to be resilient against heat waves.