Shall We Only Store Popular Products? Warehouse Assortment Selection for E-Companies.
By Dr Li Xiaobo
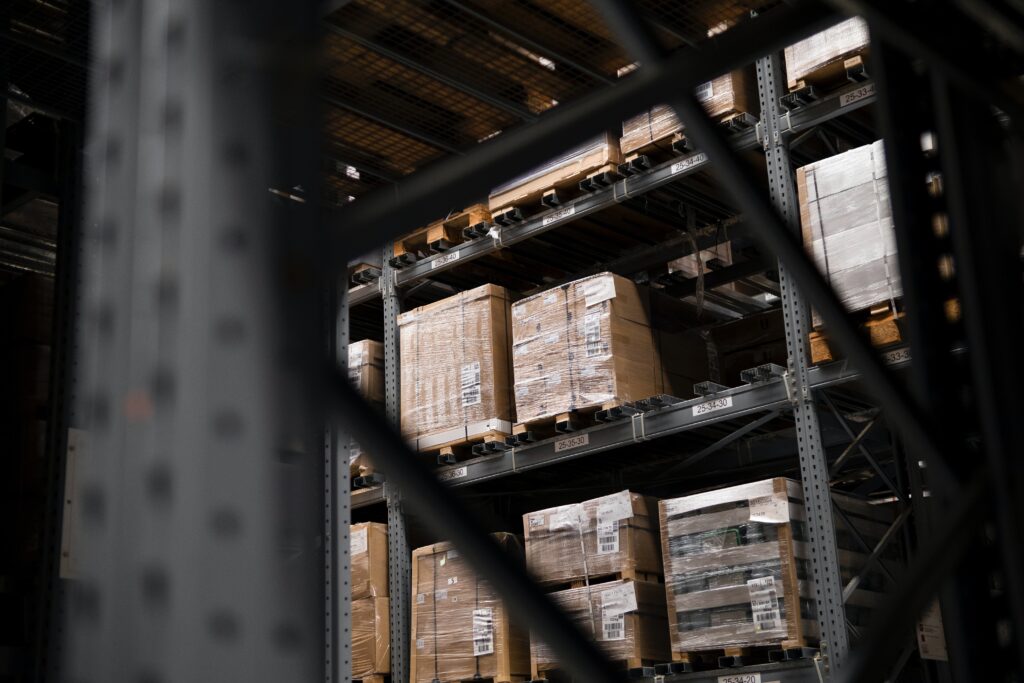
E-commerce has grown significantly in recent years, leading to an increased focus on fast and efficient delivery. Many online retailers, such as Amazon, Alibaba, and JD.com, offer same-day or even two-hour delivery options, which require the operation of warehouses in urban areas close to customers. This can make it challenging to choose the right assortment of products to store in these warehouses, as inappropriate selection can lead to increased fulfilment costs and delays, negatively impacting a company's profits and customer satisfaction. JD.com, for example, uses a two-tier distribution system in which local distribution centers serve their local areas and regional distribution centers support both their local areas and the corresponding local centers.
The main goal of selecting the product assortment for a local warehouse is to fulfil customer orders with the least fulfilment cost. When a customer places an order, the cost of fulfilling it is the lowest when the local warehouse can fully meet the order. However, if the local warehouse cannot fully cover the order, it is either fulfilled by a central warehouse through spillover fulfilment or split into multiple suborders that are fulfilled by other local warehouses, which can result in additional shipment and operational costs and higher fulfilment delay.
This paper examines the single-warehouse assortment selection problem, which aims to minimize the order fulfilment cost while maintaining a certain number of products. We propose two cost functions related to fulfilment, one for spillover fulfilment and one for order-splitting. We show that the objective function is submodular, which allows us to use submodular function optimization techniques for this problem. However, we find that the problem belongs to the NP-Hard class, and cannot be solved in polynomial time. Despite this, we are able to formulate the problem as a mixed integer linear program, which can be efficiently solved by solvers such as Gurobi.
We also propose a simple heuristic called the marginal choice indexing (MCI) policy to solve the warehouse assortment problem. The MCI policy selects the K products with the highest marginal choice probability to store in the warehouse. While the MCI policy may not perform well in extreme cases, we identify sufficient conditions on the customer demand distribution for the MCI policy to be optimal. Through numerical experiments using synthetic data, we find that the MCI policy has good average performance and is robust when the actual demand distribution is not known. In fact, under certain circumstances, the MCI policy may outperform the MILP optimal solution based on observed demand.
We conducted extensive numerical experiments on a real-world dataset from RiRiShun Logistics and found that the MCI policy is near-optimal in all the settings we tested. By applying the MCI policy, the fill rate is estimated to improve by an average of 9.18% compared to the current practice for local transfer centers on the training dataset. Moreover, the MCI policy outperformed the MILP optimal solution in 14 out of 25 cases on the test dataset, highlighting its robustness against the noise of demand estimation. These positive numerical results suggest that the techniques developed in this paper can be effectively applied in practice.
This paper is a joint work with Lin Hongyuan and Liu Fang. It won the 2022 INFORMS MSOM Data Driven Research Challenge.